The Role of AI and Machine Learning in Predicting Sports Bets
The world of sports betting has witnessed a significant transformation with the emergence of artificial intelligence (AI) and machine learning. These technologies have revolutionised the way predictions are made, offering bettors powerful tools to analyse data, identify patterns, and make informed decisions. This article explores the role of AI and machine learning in predicting sports bets, highlighting their advantages, implementation strategies, predictive factors, limitations, ethical considerations, successful applications, and future trends.
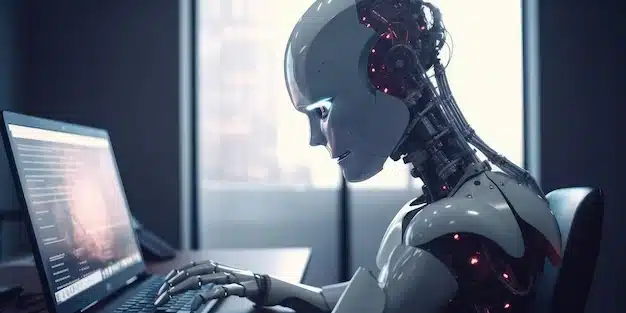
Table of Contents
Introduction to AI and Machine Learning in Sports Betting
What is AI in Sports Betting?
AI in sports betting refers to the application of advanced algorithms and computational models to analyse vast amounts of data, identify patterns, and generate predictions related to sports outcomes. It involves using intelligent systems to simulate human-like decision-making processes based on data-driven insights.
Understanding Machine Learning in Sports Betting
Machine learning is a subset of AI that focuses on enabling computers to learn and improve from experience without being explicitly programmed. In sports betting, machine learning algorithms can be trained on historical data to recognize patterns, adapt to changing conditions, and make accurate predictions.
Advantages of AI and Machine Learning in Predicting Sports Bets
The use of AI and machine learning in predicting sports bets offers several advantages over traditional methods. This section explores these advantages, highlighting their impact on the accuracy and efficiency of sports betting predictions.
Data Analysis and Pattern Recognition
AI algorithms excel at analysing large volumes of data and identifying complex patterns that may not be apparent to human analysts. By processing vast amounts of historical data, AI models can uncover valuable insights and trends that help inform betting strategies.
Efficient and Rapid Processing of Large Data Sets
One of the key strengths of AI and machine learning is their ability to handle and process massive data sets quickly. This enables sports bettors to analyse a wide range of factors, such as player performance, team statistics, and historical trends, in a timely manner to make well-informed betting decisions.
Continuous Learning and Adaptability
Machine learning models have the capacity to continuously learn and adapt as new data becomes available. By incorporating real-time information and adjusting their predictions accordingly, AI systems can provide up-to-date insights that reflect the latest conditions and variables affecting sports outcomes.
Implementing AI and Machine Learning Models in Sports Betting
This section delves into the practical aspects of implementing AI and machine learning models in sports betting. It covers the key steps involved, from data collection and preprocessing to feature selection, model training, and evaluation.
Data Collection and Preprocessing
To build accurate predictive models, sports bettors need to gather relevant and reliable data from various sources. This involves collecting information on team and player statistics, match results, injuries, weather conditions, and other factors that may influence game outcomes. Proper preprocessing techniques, such as data cleaning and normalisation, are applied to ensure the data is suitable for analysis.
Feature Selection and Engineering
In machine learning, feature selection is crucial to identify the most informative variables that contribute to accurate predictions. Sports bettors need to carefully choose the relevant features and may also engineer new features based on domain knowledge and insights gained from data analysis.
Model Training and Evaluation
Once the data is prepared, machine learning models are trained using historical data to identify patterns and relationships between input features and outcomes. The models are then evaluated using appropriate performance metrics to assess their predictive capabilities and ensure their reliability.
Predictive Factors and Metrics Used in AI Sports Betting Models
This section explores the predictive factors and metrics commonly used in AI sports betting models. It highlights the key elements that AI algorithms consider when making predictions, including historical performance and statistics, team and player attributes, and external factors and contextual data.
Historical Performance and Statistics
Historical performance data, such as past match results, team standings, and individual player statistics, serve as valuable inputs for AI models. By analysing this data, machine learning algorithms can identify patterns and trends that may influence future outcomes.
Team and Player Attributes
AI sports betting models take into account various team and player attributes, including playing styles, strengths, weaknesses, injuries, and recent form. By assessing these factors, the models can estimate the impact of specific players or team dynamics on game results.
External Factors and Contextual Data
In addition to internal factors, AI models consider external factors and contextual data that may affect sports outcomes. These factors may include weather conditions, venue, crowd support, historical rivalries, and other situational variables that can influence the performance of teams and players.
Challenges and Limitations of AI and Machine Learning in Sports Betting
While AI and machine learning offer promising capabilities in predicting sports bets, they are not without challenges and limitations. This section explores some of the key hurdles that sports bettors and AI practitioners need to navigate.
Uncertainty and Variability in Sports Outcomes
Sports events are inherently unpredictable, and even the most sophisticated AI models cannot guarantee accurate predictions in all cases. The inherent variability and uncertainty in sports outcomes pose a challenge for AI systems, requiring bettors to consider probabilistic approaches rather than absolute certainties.
Overfitting and Data Bias
Overfitting occurs when a machine learning model becomes too closely fitted to the training data, resulting in poor generalisation to new data. Sports bettors must be cautious about overfitting their models and ensure that the algorithms do not become overly biassed towards specific data patterns that may not hold true in the future.
Human Factors and Expertise
While AI and machine learning algorithms can process vast amounts of data, human expertise and domain knowledge still play a vital role in sports betting. AI models may struggle to capture the subtle nuances and intangibles of sports events that human analysts can identify through their experience and understanding of the game.
Ethical Considerations in AI Sports Betting
The utilisation of AI and machine learning in sports betting raises ethical considerations that need to be addressed. This section highlights important ethical aspects, including responsible gambling, fairness and transparency in model algorithms, and privacy and data protection.
Responsible Gambling and Addiction Prevention
AI sports betting models should be used responsibly, promoting responsible gambling practices and mitigating the risk of addiction. It is essential to emphasise that AI predictions are not guarantees of success and that individuals should gamble within their means and with a clear understanding of the associated risks.
Fairness and Transparency in Model Algorithms
AI models used in sports betting should be fair and transparent. Bettors and AI practitioners need to ensure that the algorithms and decision-making processes are free from bias, discrimination, or hidden agendas. Transparency in model algorithms builds trust among users and allows for independent evaluation.
Privacy and Data Protection
As AI systems rely on vast amounts of data, including personal and sensitive information, privacy and data protection become crucial considerations. It is important to handle and store data securely, respecting privacy regulations and obtaining necessary consents when collecting personal information for AI sports betting purposes.
Successful Applications of AI and Machine Learning in Sports Betting
This section showcases the successful applications of AI and machine learning in sports betting. It explores various areas where AI has made a significant impact, including sports betting prediction platforms, odds analysis, line movement prediction, and real-time predictions during live betting.
Sports Betting Prediction Platforms
AI-powered sports betting prediction platforms leverage machine learning models to generate predictions and recommendations for bettors. These platforms utilise advanced algorithms and historical data to provide users with insights, statistical analysis, and betting suggestions to inform their decision-making.
Odds Analysis and Line Movement Prediction
AI algorithms can analyse odds and line movements in sports betting markets to identify favourable betting opportunities. By detecting patterns and anomalies, machine learning models can help bettors understand the market dynamics and make informed decisions based on changes in betting lines and odds.
Live Betting and Real-Time Predictions
Real-time predictions during live betting have become increasingly popular, and AI plays a crucial role in providing timely and accurate insights. By analysing live data, including in-game statistics and situational variables, AI models can generate predictions and recommendations for bettors to capitalise on dynamic betting opportunities.
Future Trends and Developments in AI Sports Betting
The future of AI and machine learning in sports betting holds exciting possibilities. This section discusses potential trends and developments, including advancements in data collection and integration, enhanced model algorithms and techniques, and the integration of AI with sports analytics.
Advancements in Data Collection and Integration
As technology continues to evolve, sports bettors will have access to even more comprehensive and real-time data. Advancements in data collection methods, such as player tracking, sensor technologies, and advanced analytics, will provide a richer and more detailed dataset for AI models to leverage.
Enhanced Model Algorithms and Techniques
Ongoing research and development in AI and machine learning will lead to the refinement and enhancement of model algorithms and techniques. This includes the integration of advanced deep learning architectures, ensemble methods, and reinforcement learning, enabling more accurate and robust predictions in sports betting.
Integration of AI with Sports Analytics
The integration of AI with sports analytics will further enhance the capabilities of sports betting predictions. By combining AI-driven betting models with advanced sports analytics, including player performance analysis, game strategies, and opponent scouting, bettors will have access to a more comprehensive and holistic approach to sports betting.
Conclusion
AI and machine learning have revolutionised the field of sports betting, offering powerful tools for predicting outcomes and making informed betting decisions. By leveraging data analysis, pattern recognition, and continuous learning, AI systems enhance the accuracy and efficiency of sports betting predictions. However, challenges related to uncertainty, overfitting, and human expertise need to be navigated, and ethical considerations must be addressed. With responsible usage and ongoing advancements, AI and machine learning will continue to shape the future of sports betting, enabling more accurate predictions and an enhanced betting experience.